New Paper on "Neural network based rate- and temperature-dependent Hosford-Coulomb fracture initiation model"
Our new paper on "Neural network based rate- and temperature-dependent Hosford-Coulomb fracture initiantion model" was publish in International Journal of Mechanical Sciences
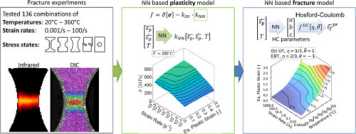
external pageplease refer to Sciencedirect to review the full article
Abstract
The accurate description of the strain rate and temperature dependent response of metals is a perpetual quest in crashworthiness and forming applications. In the present study, experiments are carried out to probe the onset of ductile fracture for an aluminum alloy AA7075-T6 for 136 combinations of stress state, strain rate and temperature. The experimental campaign covers strain rates ranging from 0.001/s to 100/s, and temperatures ranging from 20 °C to 360 °C. We combine a YLD2000 yield surface with a neural network based hardening law to describe the external pagelarge deformation plasticity response of the material. The NN-based hardening law is trained on experimental data, achieving 3.9% accuracy on force predictions including the post-necking regime. The loading paths to fracture are extracted for each simulation, showcasing non-proportionally evolving stress triaxiality, Lode angle parameter, strain rate and temperature. A neural network parameterized Hosford–Coulomb fracture locus is proposed, which is trainable using these evolving loading histories. The accuracy of the proposed fracture model is validated against the experimental onset of fracture, predicting the fracture onset at an error of 8%.