New Paper on "Transfer learning of recurrent neural network-based plasticity models"
Our new paper on "Transfer learning of recurrent neural network-based plasticity models" was published in International Journal for Numerical Methods in Engineering.
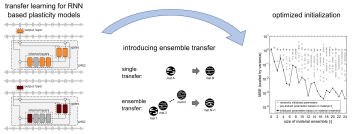
external pagePlease refer to Sciencedirect to review the full article
Abstract
Mechanics-specific recurrent neural network (RNN) models are known for their ability to describe the complex three-dimensional stress–strain response of elasto-plastic solids for arbitrary loading paths. To apply RNN models to real materials, it is crucial to identify a strategy that allows for their training from small datasets that could be obtained from robot-assisted experiments. It is demonstrated that regular training with datasets comprising random walks (RWs) in strain space yield a significantly higher generalization ability than the same number of sequences for smooth loading paths. Moreover, it is found that transfer learning, that is, initializing the weights and biases with the parameters from an already trained material, improves the convergence rates and reduces the required number of stress–strain sequences for training. When leveraging the experience gained for multiple materials through ensemble transfer learning, even more substantial improvements are obtained. For example, the same model accuracy and generalization ability is obtained from training with 400 smooth stress–strain sequences after ensemble transfer as from training with 10,000 RW sequences after regular training.