New Paper on Recurrent neural network plasticity models
Our new paper on "Recurrent neural network plasticity models: Unveiling their common core through multitask learning" was published in Computer Methods in Applied Mechanics and Engineering
Abstract
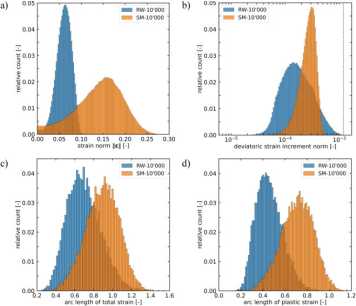
Recurrent neural network models are known to be particularly suitable for data-driven constitutive modeling due to their built-in memory variables. The main challenge preventing their widespread application to engineering materials lies in their excessive need of data for training. Here, we postulate that RNN models of elasto-plastic solids feature a large common core that is shared by all materials of the same class. The common core is complemented by material-specific layers with parameters that vary from material-to-material. After training RNN models for 25 different von Mises materials, we identify the common core of a multi-task model. Furthermore, we demonstrate through ensemble transfer learning that adding a new material to the multi-task model requires datasets that are two to three orders of magnitude smaller than the datasets needed for training an RNN from scratch. In addition, to multi-task learning, we introduce probabilistic ensembles of RNN plasticity models to quantify the epistemic uncertainty. A deep drawing simulation is performed to demonstrate the superior generalization capabilities of RNNs identified via multi-task learning as compared to those obtained through single task training.